Recent breakthroughs in generative AI have centered largely on language and imagery—from chatbots that compose sonnets and analyze text to voice models that mimic human speech and tools that transform prompts into vivid artwork. But global chip giant Nvidia is now making a bolder claim: the next chapter of AI is about systems that take action in high-stakes, real-world scenarios.
At the recent International Conference on Learning Representations (ICLR 2025) in Singapore, Nvidia unveiled more than 70 research papers showcasing advances in AI systems designed to perform complex tasks beyond the digital realm.
Driving this shift are agentic and foundational AI models. Nvidia’s latest research highlights how combining these models can influence the physical world—spanning adaptive robotics, protein design, and real-time reconstruction of dynamic environments for autonomous vehicles. As demand for AI grows across industries, Nvidia is positioning itself as a core infrastructure provider powering this new era of intelligent action.
Bryan Catanzaro, vice president of applied deep learning research at Nvidia, described the company’s new direction as a full-stack AI initiative.
“We aim to accelerate every level of the computing stack to amplify the impact and utility of AI across industries,” he tells Fast Company. “For AI to be truly useful, it must evolve beyond traditional applications and engage meaningfully with real-world use cases. That means building systems capable of reasoning, decision-making, and interacting with the real-world environment to solve practical problems.”
Among the research presented, four models stood out—one of the most promising being Skill Reuse via Skill Adaptation (SRSA).
This AI framework enables robots to handle unfamiliar tasks without retraining from scratch—a longstanding hurdle in robotics. While most robotic AI systems have focused on basic tasks like picking up objects, more complex jobs such as precision assembly on factory lines remain difficult. Nvidia’s SRSA model aims to overcome that challenge by leveraging a library of previously learned skills to help robots adapt more quickly.
“When faced with a new challenge, the SRSA approach analyzes which existing skill is most similar to the new task, then adapts and extends it as a foundation for learning,” Catanzaro says. “This brings us a significant step closer to achieving generalization across tasks, something that’s crucial for making robots more flexible and useful in the real world.”
To make accurate predictions, the system considers object shapes, movements, and expert strategies for similar tasks. According to one research paper, SRSA improved success rates on unseen tasks by 19% and required 2.4 times fewer training samples than existing methods.
“Over time, we expect this kind of self-reflective, adaptive learning to be transformative for industries like manufacturing, logistics, and disaster response—fields where environments are dynamic and robots need to quickly adapt without extensive retraining,” Catanzaro says.
Biotech breakthroughs
The biotech sector has traditionally lagged in adopting cutting-edge AI, hindered by data scarcity and the opaque nature of many algorithms. Protein design, essential to drug development, is often hampered by proprietary data silos that slow progress and stifle innovation.
To address this, Nvidia introduced Proteína—a large-scale generative model for designing entirely new protein backbones. Built using a powerful class of generative models, it can produce longer, more diverse, and functional proteins—up to 800 amino acids in length. Nvidia claims it outperforms models like Google DeepMind’s Genie 2 and Generate Biomedicines’ Chroma, especially in generating large-chain proteins.
According to a paper on Proteína, the team trained the model using 21 million high-quality synthetic protein structures and improved learning thanks to new guidance strategies that ensure realistic outputs during generation. This breakthrough could transform enzyme engineering (and, by extension, vaccine development) by enabling researchers to design novel molecules beyond what occurs in nature.
“What makes it especially powerful is its ability to generate proteins with specific shapes and properties, guided by structural labels,” Catanzaro says. “This gives scientists an unprecedented level of control over the design process—allowing them to create entirely new molecules tailored for specific purposes, like new medicines or advanced materials.”
A new AI tool for autonomous vehicles
Another standout from ICLR 2025 is Spatio-Temporal Occupancy Reconstruction Machine (STORM), an AI model capable of reconstructing dynamic 3D environments—like city streets or forest trails—in under 200 milliseconds. With minimal video input, it produces detailed, real-time spatial maps that can inform rapid machine decision-making. Nvidia sees STORM as a tool for autonomous vehicles, drones, and augmented reality systems navigating complex, moving environments.
“One of the biggest backlogs in current models is that they often rely heavily on optimization—an iterative process that takes time to refine and produce accurate 3D reconstructions,” says Catanzaro. “STORM tackles this by achieving high-accuracy results in a single pass, significantly speeding up the process without sacrificing quality.”
STORM’s potential extends beyond vehicles. Catanzaro envisions applications in consumer tech, such as AR glasses capable of mapping a live sports game in real time—allowing viewers to experience the event as if they were on the field. “STORM’s real-time environmental intelligence moves us closer to a future where machines and devices can perceive, understand, and interact with the physical world as fluidly as humans do,” he says.
While STORM is built to help machines understand the physical world in real time, Nvidia is also pushing the boundaries of how large language models reason—through a project called Nemotron-MIND. This 138-billion-token synthetic pretraining data set is designed to enhance both mathematical and general reasoning. At its core is MIND, a new framework that turns raw math-heavy web documents into rich, multi-turn conversations that mirror how humans work through problems together.
By turning dense math documents into conversations between people with different levels of understanding, MIND helps AI models break down problems step by step and explain them naturally. This method doesn’t just teach models what the right answer is—it helps them learn how to think through problems like a person would.
According to its research paper, a seven-billion-parameter model trained on just four billion tokens of MIND-style dialogue outperformed much larger models trained on traditional data sets. It showed significant gains on key reasoning benchmarks like GSM8K (grade school math), MATH, and MMLU (massive multitask language understanding), and achieved a 2.5 percent boost in general reasoning when integrated into an LLM.
Can startups and researchers keep up?
Training and deploying advanced AI models requires substantial GPU resources, often out of reach for smaller players. To close this gap, Nvidia is rolling out its next-gen AI models through Nvidia Inference Microservices (NIMs), a suite of containerized, cloud-native tools designed to simplify deployment across different infrastructures. NIM includes prebuilt inference engines for a wide array of models, helping organizations integrate and scale AI with fewer computing resources.
“Improving efficiency has always been a major focus for us,” Catanzaro says. “Ultimately, our goal is to democratize access to AI capabilities and make deployment practical at every scale, regardless of their computing resources, to harness the power of AI.”
As agentic and foundational AI becomes more capable and more embodied, the future of tech may hinge on how effectively it works with humans. “It’s critical to identify and support use cases across diverse fields,” Catanzaro says.
Accedi per aggiungere un commento
Altri post in questo gruppo
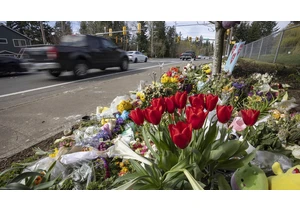

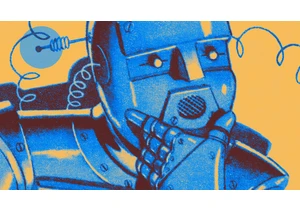
Since ChatGPT sparked the generative AI revolution in November 2022, interacting with AI has felt like using a digital confession booth—private, intimate, and shielded from public view (unless you

Live and on-demand video constituted an estimated 66% of global internet t
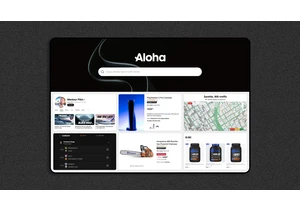
My worst workday habit is that I’m a compulsive web page checker.
Throughout the day, I’m constantly refreshing the same handful of sites for updates. I’ll check the me

Cloud storage services conveniently let you store and access documents, photos, videos, and more from any device. The best part? Many top providers offer free plans that are surprisingly capable.

Sometimes, you need to shake things up in your career. Maybe the job isn’t as fulfilling anymore. Maybe changing circumstances are pushing you toward a new path. Either way, figuring out what to d